The proliferation and democratization of Artificial Intelligence has fueled the growth of IoT analytics. Data collected from IoT devices—images, videos, sensor data, and time-series data—are used to train Machine Learning (ML) models, generating valuable new insights into the IoT overall. However, these applications require powerful and expensive solutions, either in the cloud or on on-premises servers and gateways that rely on complex chipsets.
At the same time, Edge AI chipsets have extended AI to countless end points, including mobile devices, automobiles, smart home speakers, and wireless cameras. However, these devices struggle to support high computing performance and high data throughput, causing latency issues, which is a death knell for AI.
TinyML aims to solve the issues of both cost and power efficiency by enabling data analytics performance on low-powered hardware with low processing power and small memory size, aided by software designed for small-sized inference workloads. It has the potential to revolutionize the future of the IoT.
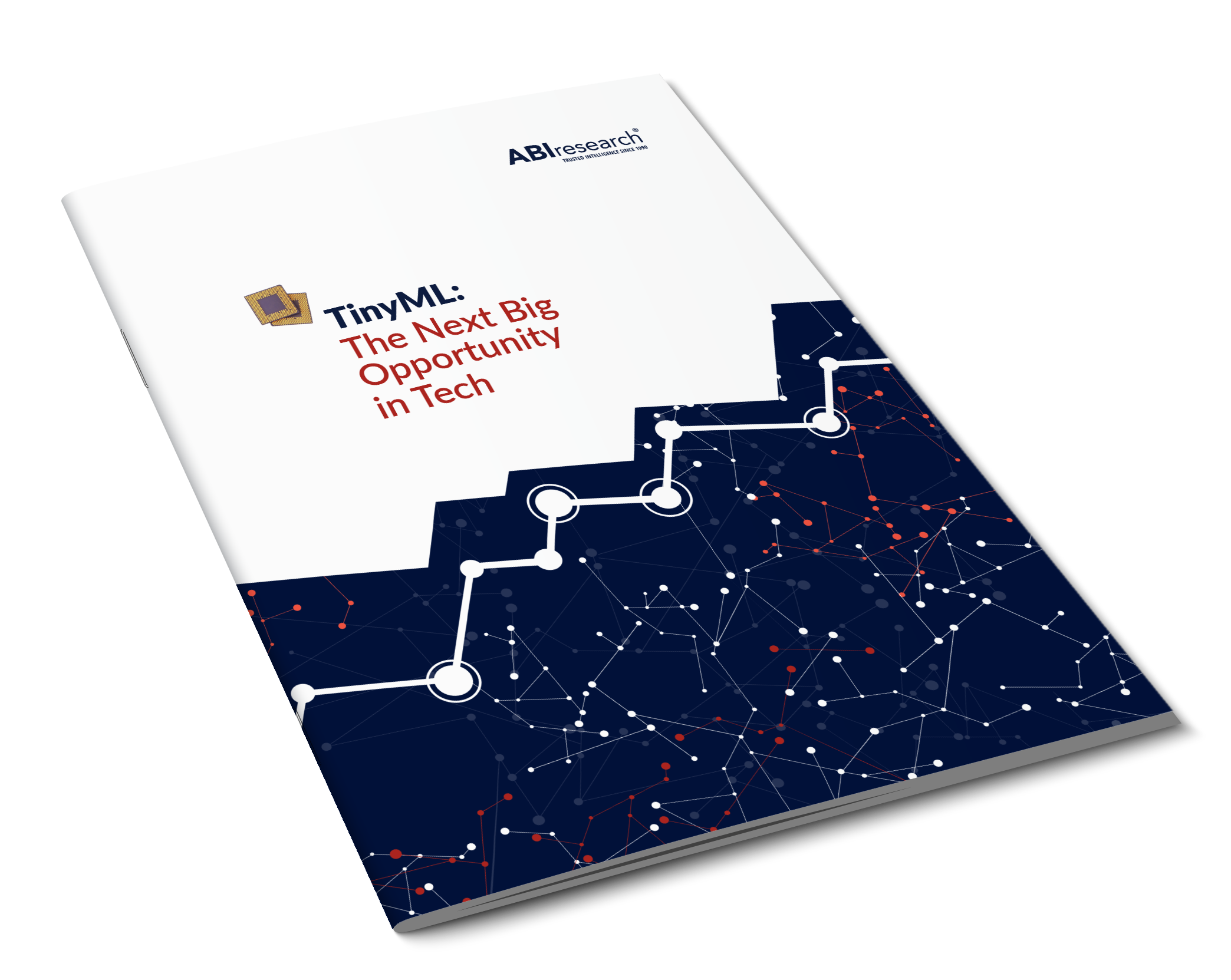